Synthetic Data: The Future of AI Model Training – Best Tech 2025
Synthetic Data: The Future of AI Model Training
Synthetic Data: The Future of AI Model Training: The field of artificial intelligence (AI) is being pursued along various paths, revolutionizing industries like healthcare, finance, and more. Synthetic data offers a groundbreaking approach to overcoming challenges associated with traditional datasets, particularly in privacy-sensitive sectors. From generating realistic human faces to building robust machine learning models, synthetic data is proving to be a vital tool for AI scalability and innovation.
Understanding
Unlike traditional datasets, which are often sourced from actual events or entities, synthetic datasets are created using algorithms and simulations. This capability makes synthetic data a valuable resource for developers who need privacy-compliant data for training AI models.
The artificial intelligence cycle—spanning data collection, model training, validation, and deployment—relies heavily on high-quality data. Synthetic data provides a scalable solution for feeding this cycle without the constraints of privacy concerns, data scarcity, or regulatory limitations.
Why Synthetic Data Matters
Privacy-Compliance and Ethical AI: Traditional methods of anonymizing data often fall short of meeting stringent regulations like HIPAA in healthcare or GDPR in the European Union. Synthetic data bridges this gap by eliminating identifiable elements while preserving the statistical properties of real data. Training on synthetic data enables developers to build AI models without exposing sensitive information, ensuring compliance with privacy laws.
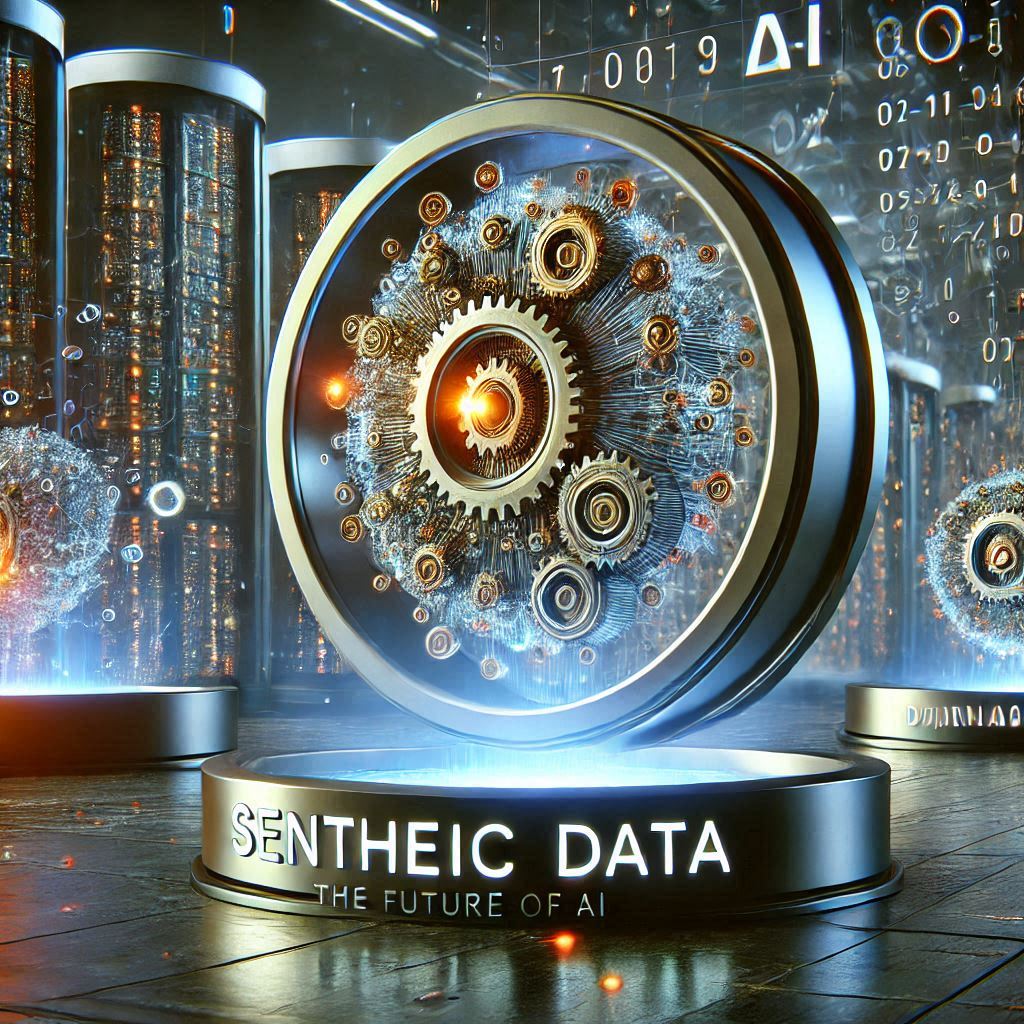
Scalability for AI Model Training: One of the challenges of AI model training is obtaining large, diverse, and representative datasets. Synthetic data offers an efficient way to scale data generation. With tools and frameworks for creating synthetic datasets, developers can generate limitless variations tailored to specific use cases. This scalability accelerates the development process and enhances AI’s predictive capabilities.
Enhanced Performance for Machine Learning Models: Synthetic data can augment existing datasets, addressing biases and filling gaps in data coverage. For instance, generative AI can simulate rare medical conditions or financial fraud scenarios, which are difficult to capture in traditional datasets. By training AI models on these
enriched datasets, developers can achieve greater accuracy and robustness in their predictions.
Applications of Synthetic Data
Autonomous Vehicles: The development of self-driving cars relies heavily on synthetic datasets to simulate diverse driving scenarios, weather conditions, and road environments. discovery & personalized medicine & diagnostics.For example, synthetic training data can be used to model complex diseases without compromising patient privacy.
Privacy-compliant data training ensures sensitive financial transactions remain secure.
Retail and Marketing: synthetic data sets allow businesses to analyze consumer behavior and optimize marketing strategies without relying on invasive tracking methods.
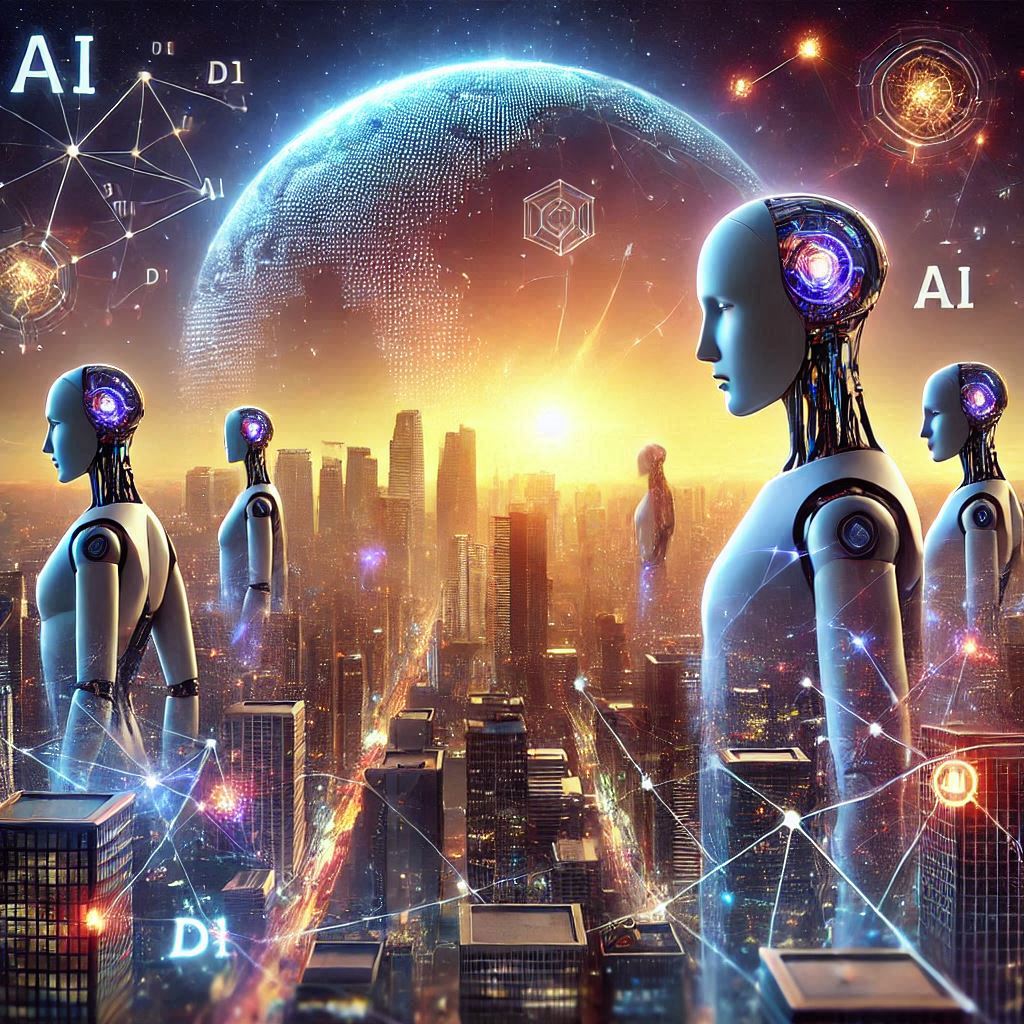
Synthetic Data: Transforming the AI Landscape
The rise of machine learning, AI, and data science has brought synthetic data to the forefront of AI innovation. As AI models evolve, the demand for high-quality, diverse training data continues to grow. Synthetic data for AI training offers a pathway to address this need while maintaining ethical and privacy standards.
Developers are exploring tools and techniques for how to create synthetic data from real data. These methods include generative adversarial networks (GANs), which can create hyper-realistic images, and simulation software for generating contextual data.
Challenges
Additionally, widespread adoption of synthetic training data requires a cultural shift within organizations to trust artificial data sources.
Looking ahead, the future in AI and machine learning is intertwined with synthetic data innovations. Open-source tools and freeware AI platforms are making it easier for smaller organizations to adopt this technology. Moreover, research into the artificial intelligence cycle and machine learning AI data science continues to refine ssynthetic data generation techniques.
Conclusion
By offering a privacy-compliant, scalable, and efficient solution, synthetic data is bridging the gap between data scarcity and the growing demands of AI applications.
The benefits of training on synthetic data, combined with advancements in generative AI and machine learning, ensure that synthetic data will remain at the forefront of AI innovation. As organizations embrace synthetic AI training data, they unlock new possibilities in data science, pushing the limits of what AI can achieve.
Synthetic Data: The Future of AI Model Training is no longer a futuristic concept; it’s a reality shaping the AI-driven world today.
Synthetic Data:
Key Opinion: Synthetic data provides a privacy-compliant and scalable solution for training AI models, pushing the boundaries of what’s possible in data science.
Focus Words: Synthetic data, AI model training, data privacy, healthcare AI, scalable data solutions
SEO Keywords: synthetic data for AI, privacy-compliant data training, AI in healthcare, synthetic datasets, AI scalability
Using Keywords: generative ai, machine learning, ai and the future, machine learning what is, future with ai, ai and future, future in ai, future for ai, freeware ai, and machine learning, machine learning and, machine learning machine learning, synthetic data
machine learning ai & data science, ai models course, synthetic training data, synthetic ai training data
artificial intelligence cycle,